How to cite: DataStream Initiative (2022). DataStream Data Governance Policy. DataStream.org/en-ca/documentation/data-policy
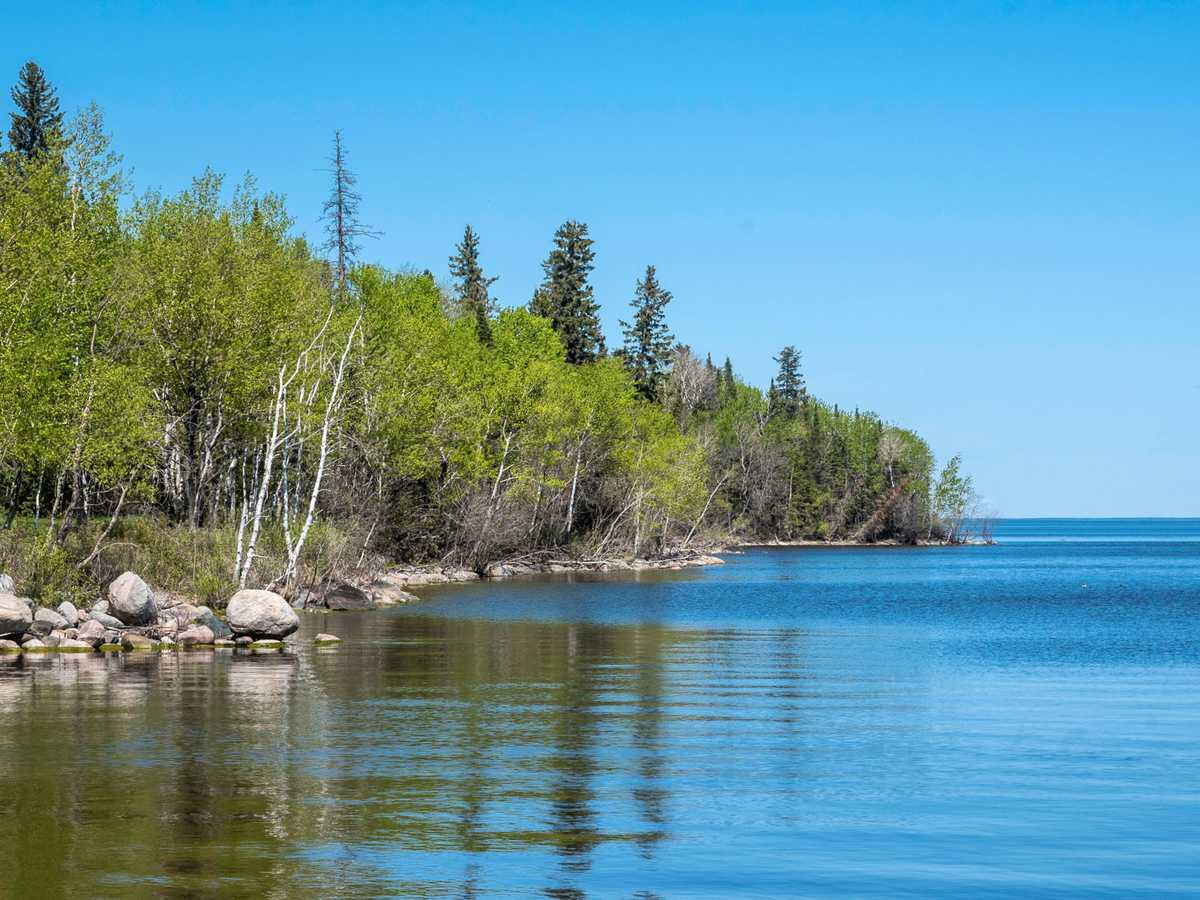
DataStream
Data Governance Policy
This policy sets out high level principles for how DataStream’s open data platform is delivered to ensure the greatest benefit and value to all users and contributors.
About DataStream
DataStream is an open access platform for sharing Western scientific water and sediment quality data. Our mission is to promote knowledge sharing and advance collaborative water stewardship, so our waters remain healthy for generations to come.
DataStream works with water monitoring initiatives and organizations of all kinds that want to share their data publicly – in secure, accessible, and standardized formats. Data contributors maintain ownership of their data and control over what they choose to publish on the system.
Guiding Principles
The principles outlined below set the foundational values and concepts that guide the ongoing delivery of DataStream’s open data platform, and the approach to storing and managing the data contained within it.
- Open Access
- Accessibility
- Data Quality
- Interoperability
- Data Security
- Sustainability
These principles are informed by evolving national and international best practices, including those articulated within the FAIR principles for scientific data management (Findable, Accessible, Interoperable, Reusable) [1], CARE principles for Indigenous data governance (Collective benefit, Authority to Control, Responsibility, Ethics) [2], the First Nations principles of OCAP® (Ownership, Control, Access and Possession) [3], and the TRUST principles for digital repositories (Transparency, Responsibility, User focus, Sustainability) [4].
1. Open Access
Data are made openly available on an equal basis, freely and in a timely way.
Making data broadly available without restriction (open access) is a growing movement worldwide. It is particularly relevant for environmental data and data collected in the public interest, using public funds. Open data supports stronger science, enhances transparency in decision-making and facilitates collaboration among people and organizations.
Making data open involves minimizing or eliminating barriers to data access and use (such as the use of restrictive data sharing agreements, proprietary file formats or high-cost tools). Open data is digital data that is made available with the technical and legal characteristics necessary for it to be freely used, reused and redistributed by anyone, subject at most to the requirement of providing attribution. For data to meet the definition of being ‘open’, it needs to be shared in a non-proprietary, structured, machine-readable format, and with clear licencing that permits reuse.
What DataStream is doing...
- DataStream is free and open for anyone to use; access to data is not tiered or password protected
- All datasets on DataStream are published under open data licenses, which provide clarity around data ownership, attribution and reuse
- All data can be downloaded in structured .csv files (a machine readable, non-proprietary format), or accessed via an API
- Every water monitoring observation (data point) is accompanied by sufficient metadata on download to make it usable and/or assess fitness for use in a given context (e.g. location information, units of measurement, lab analysis method and detection limit)
- Dataset Digital Object Identifiers (DOI)s facilitate data citation and ensure findability over the long-term by preventing broken links
2. Accessibility
Design and deliver DataStream in ways that reduce barriers to participation.
Digital accessibility is the practice of creating websites and web tools so they can be used by as many people as possible, including those with disabilities, slow or limited internet access, and varying levels of technical and digital literacy. This involves making sure websites and apps are appropriately designed and coded, applying relevant accessibility standards and guidelines, and continually improving the user experience for everyone. Doing so reduces barriers to participation, whether they be physical, geographic, cultural, linguistic, digital, financial or other.
What DataStream is doing…
- Webpages can be accessed in both English and French, and are navigable by keyboard
- Data can be explored through map-based search, interactive data visualizations and science explainer material (in addition to .csv file download or API access)
- Website testing is conducted with a simulated slow mobile connection to ensure the site works well for those with slow or limited internet access
- Resource library includes how-to videos, text-based guidance documents, recorded webinars and information sessions
- Tailored onboarding support for data contributors
- Closed-captioning of videos and inclusion of “alt text” for images
3. Data Quality
Strive for completeness of datasets and adherence to widely adopted data standards that support data reuse.
Efforts are needed to ensure datasets are complete and quality controlled so that data are reliable and can confidently be used to better understand water quality across watersheds. Established and well-defined data standards with consistent vocabularies protect against ambiguity in data reporting and ensure the appropriate metadata (data that provides context or additional information about the data) is included. This facilitates the reuse, interpretation, and appropriate aggregation of data collected by diverse entities.
The metadata that accompanies datasets should adhere to existing standards for cataloguing data and evaluating fitness for purpose. Data should also be linked with related datasets, if any exist, in order to show relationships among initiatives and datasets.
What DataStream is doing…
- All data on DataStream is shared in a standardized format based on the WQX schema for the exchange of water quality data (developed by the US EPA and USGS)
- Schema validation checks during the data upload process ensure conformity with the DataStream-WQX data schema and that all necessary information is included (e.g., sample location, units of measurement, analysis method and detection limits for lab data, etc.)
- Quality Control warnings during the upload process indicate when results or other attributes are outside of expected values (e.g., a pH value outside of 0-14)
- Dataset metadata is accessible in DCAT (json, xml), ISO 19115
- Version control and dataset changelog document updates to a dataset over time
4. Interoperability
Strive for technological and semantic interoperability with other initiatives.
The digital infrastructure ecosystem is dependent upon the cooperative flow of data across platforms. This movement towards widespread cooperation and exchange has the potential to help address complex, large-scale scientific problems and environmental challenges.
A prerequisite for such cooperation is ‘interoperability’. Interoperability allows diverse systems and entities to work together (inter-operate) towards shared objectives. Interoperability has both technological and human elements. From a technological standpoint, interoperability involves, among other things, releasing data in open, machine-readable, standardized formats. The human and organizational side of interoperability requires consistent communication among participants and engagement at key decision-making junctures to inform the evolution of the system.
Sustaining interoperability requires a high level of flexibility to adapt with the rapid and often unpredictable changes in information technology, the characteristics of various research approaches and cultural diversity within and across regions.
What DataStream is doing…
- To improve data discoverability, DataStream is integrated with data catalogues and indexing systems, including Google Dataset Search, Canada’s Federated Research Data Repository (FRDR), DataCite, POLDER Federated Search, and Canadian Integrated Ocean Observing System (CIOOS).
- To improve data flows, DataStream is integrated with other data platforms including open government portals and community science platforms like Water Rangers
- Implementation of widely-adopted data and metadata standards, including alignment with how data is reported in the US Water Quality Portal (i.e. WQX data schema)
- DataStream’s API allows other tools (like data analysis and synthesis tools) to pull data directly from DataStream, for example SEAGULL (Great Lakes Observing System) and WWF Watershed Reports
5. Data Security
Safeguard the integrity and security of data against corruption and loss to ensure fitness for use over the short and long-term.
The rapid pace of information technology development has brought about new cybersecurity challenges. To facilitate trust and safeguard investments in data collection, management and sharing, it is essential that data published and stored in repositories be protected from corruption and loss over time. Cybersecurity best practices, including strong cryptography and permissions, ensure data is immutable (protected from accidental or malicious alteration or destruction).
What DataStream is doing…
- Implementation of industry security best practices from the ground up, with continuous monitoring and updates as these best practices evolve
- Blockchain technology is used to verify that data accessed from DataStream is the same data that a contributor uploaded
6. Sustainability
Support the ongoing maintenance and improvement of DataStream, and ensure long-term preservation of data stored within it.
To remain viable and functional over time, data systems require ongoing maintenance and updates to keep pace with evolving web technologies and to meet user-needs. This requires planning for and securing adequate resourcing over the short and long-term. To safeguard data over time, including in the event of unforeseen circumstances, a data preservation plan is in place that maps out processes for ensuring continued access to data for current and future generations.
What DataStream is doing…
- Annual independent third-party code review
- DataStream is supported by a diversity of funding sources including governments and foundations. This includes DataStream’s founding donor, The Gordon Foundation, which is invested in the long-term sustainability of the system.
- Ongoing collection and evaluation of user feedback to guide platform improvements
- Member of NDRIO-Portage CoreTrustSeal Certification Support Cohort (2021-22)
Further Reading
These guiding principles were informed by a range of initiatives and publications pertaining to open data, Indigenous data governance, and data management best practices for scientific data.
Indigenous Data Governance
CARE Principles of Indigenous Data Governance. Global Indigenous Data Alliance. https://www.gida-global.org/care
First Nations Principles of OCAP. First Nations Information Governance Centre. https://fnigc.ca
National Inuit Strategy on Research. Inuit Tapiriit Kanatami. https://www.itk.ca/wp-content/uploads/2020/10/ITK-National-Inuit-Strategy-on-Research.pdf
Open Data
Government of Canada Directive on Open Government. https://www.tbs-sct.gc.ca/pol/doc-eng.aspx
Hacket, J., Olsen, R., and The Firelight Group. Dissemination of Open Geospatial Data Under the Open Government Licence-Canada Trough OCAP® Principles. Natural Resources Canada (2019). https://doi.org/10.4095/314977
Open Government Partnership. https://www.opengovpartnership.org
Open Knowledge Foundation. Open Data Handbook. https://opendatahandbook.org
Scientific Data Management, Sharing and Reuse
Beijing Declaration on Research Data. International Science Council, Committee on Data. https://doi.org/10.5281/zenodo.3552330
Lin, D., Crabtree, J., Dillo, I. et al. The TRUST Principles for digital repositories. Sci Data 7, 144 (2020). https://doi.org/10.1038/s41597-020-0486-7
Tri-Agency Statement of Principles on Digital Data Management. https://ic.gc.ca/eic/site/063.nsf/eng/h_83F7624E.html
Wilkinson, M., Dumontier, M., Aalbersberg, I. et al. The FAIR Guiding Principles for scientific data management and stewardship. Sci Data 3, 160018 (2016). https://doi.org/10.1038/sdata.2016.18
References
[1] Wilkinson, M., Dumontier, M., Aalbersberg, I. et al. The FAIR Guiding Principles for scientific data management and stewardship. Sci Data 3, 160018 (2016). https://doi.org/10.1038/sdata.2016.18
[2] Research Data Alliance International Indigenous Data Sovereignty Interest Group. (September 2019). “CARE Principles for Indigenous Data Governance.” The Global Indigenous Data Alliance. GIDA-global.org
[3] First Nations Information Governance Centre. The First Nations Principles of OCAP ®. https://fnigc.ca/ocap-training/
[4] Lin, D., Crabtree, J., Dillo, I. et al. The TRUST Principles for digital repositories. Sci Data 7, 144 (2020). https://doi.org/10.1038/s41597-020-0486-7